A Casual Analysis of Dreambooth and Fine-tuning Diffusion Models
Published on: 11-30-2024
An exploration of how Dreambooth leverages CLIP encoders for efficient fine-tuning of diffusion models.
Fine tuning is a critically important technique to leverage the capabilities of any large foundation model. LLMs(large language models) require fine tuning for alignment and security. They must be tuned to avoid propagating false or negative information in their responses. They also must be tuned to avoid leaking sensitive information about the specifics of the training data or even general information that could be used in dangerous settings. Prompt engineering is a bit different from fine tuning(and is something I hope to cover in a future article) but shares many of the goals of fine tuning. In general, you have this very powerful model that can achieve an incredible set of tasks and you want to point it in the correct direction, to get the most out of it, and ensure it operates with safeguards, to ensure it doesn't harm people. I like to think of foundation models as chaotic sources of energy. Fine tuning allows us to safely harvest this energy and drive useful applications with it. If you fine tune it too much, you lose a lot of the raw power of the original foundation model. But if you fine tune it too little, the energy could escape and cause even greater harm.
In the context of generative modeling for images, fine tuning remains a useful tool for leveraging unalligned foundation models. Fine tuning can often improve model performance on subdomains such as photorealism, anime, voxel art, and other niche artstyles. However it is usually individual users with limited compute and training examples that fine tune models for specific image subdomains. This makes it desirable to have a compute efficient fine tuning method that works well on limited data.
Enter Dreambooth, a fine tuning paradigm developed by Google Research that leverages the CLIP encoder of a diffusion model architecture. (If you're unfamiliar with diffusion models or CLIP, I highly recommend this article from Lil Log: https://lilianweng.github.io/posts/2021-07-11-diffusion-models/ . It is expertly written and includes intuitive visuals). Dreambooth achieves extraordinary results even when tuning data is very limited and compute is limited. How does it achieve this?
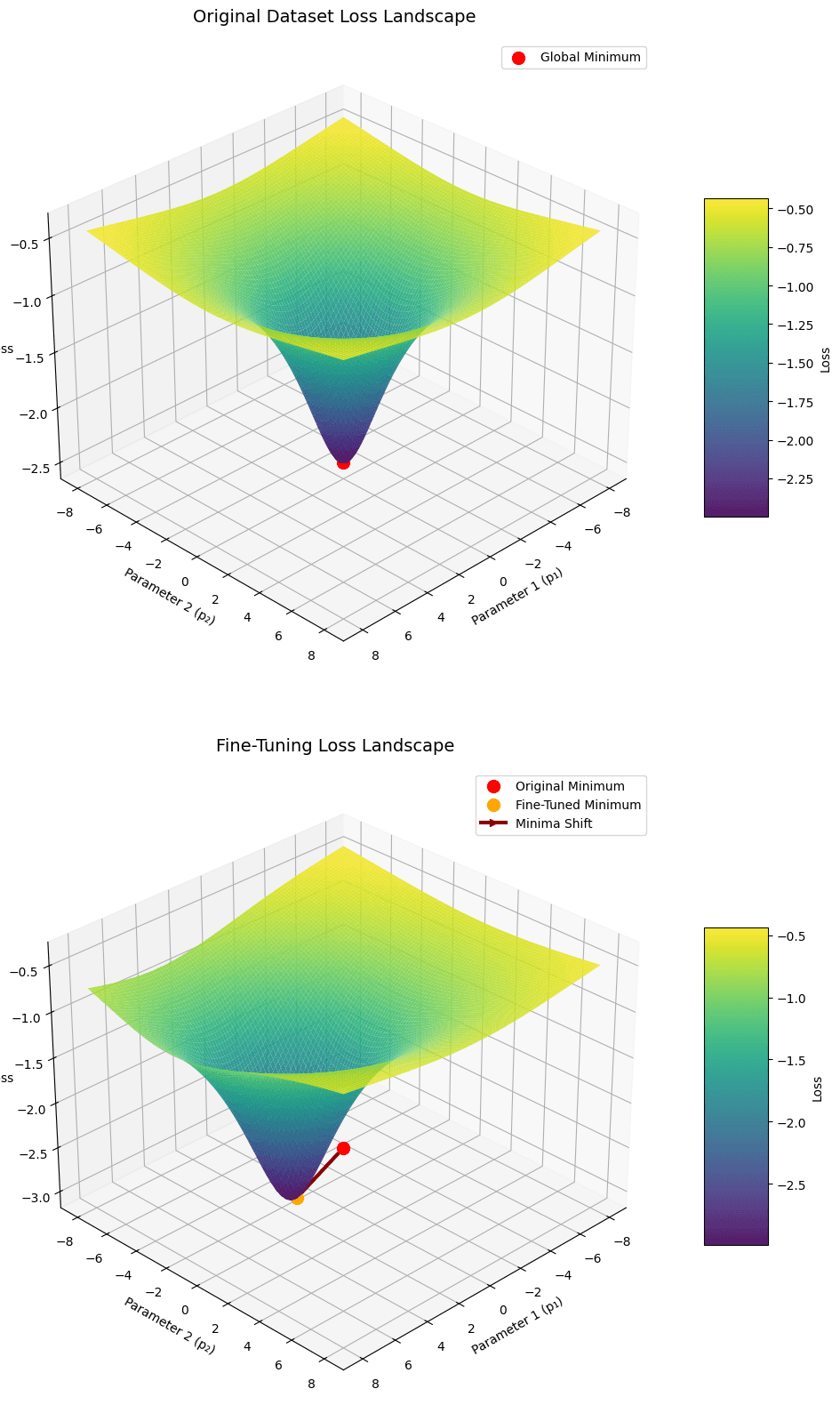
The secret sauce lies in how diffusion models encode class information. Before the advent of CLIP, diffusion models would learn classes through a simple text encoder that guided the diffusion process. This would mean that p(x_{t-1},x_t,dog) would be almost completely different from p(x_{t-1},x_t,wolf) even though these two are similar. However, CLIP leverages the relational embeddings and integrates them into the diffusion process by replacing the encoder with two encoders that generate embeddings of the image and label respectively, then use contrastive loss to minmize the distance between them.
What does this approach buy us? Most importantly, a pair of linked image and text embeddings space allows us to efficiently query for desired images. For example, take the prompt "a red ball on green grass". Without CLIP, the image results are all over the place. You get green balls on red grass, grass balls with mixes of red and green, mixes of red and green balls, etc. This is because a simple text encoder is built to only handle one class prior and can not handle complex or relational prompts. CLIP vastly increases the representational capacity of the textual input to diffusion models allowing them to precisely map language to image space.
This language to image mapping is precisely what Dreambooth takes advantage of. Instead of cumbersomely fine tuning the model and shifting the image distribution it generates, it updates the language embedder to point to different points on the existing image distribution.
With traditional fine tuning, you are retraining the model on a subset or OOD data distribution. A key assumption with fine tuning is that the optimum you are shooting for is near the optimum that the model found with the original data. That is, the model can reach the local optimum much quicker than re-training from scratch. However fine tuning model weights is a dangerous gambit. While you may increase performance on your tuning dataset, it may decrease your model capabilities overall. Your training data may not be representative of the true distribution. It could be noisy, low quality, skewed, or there may simply not be enough of it for fine tuning.
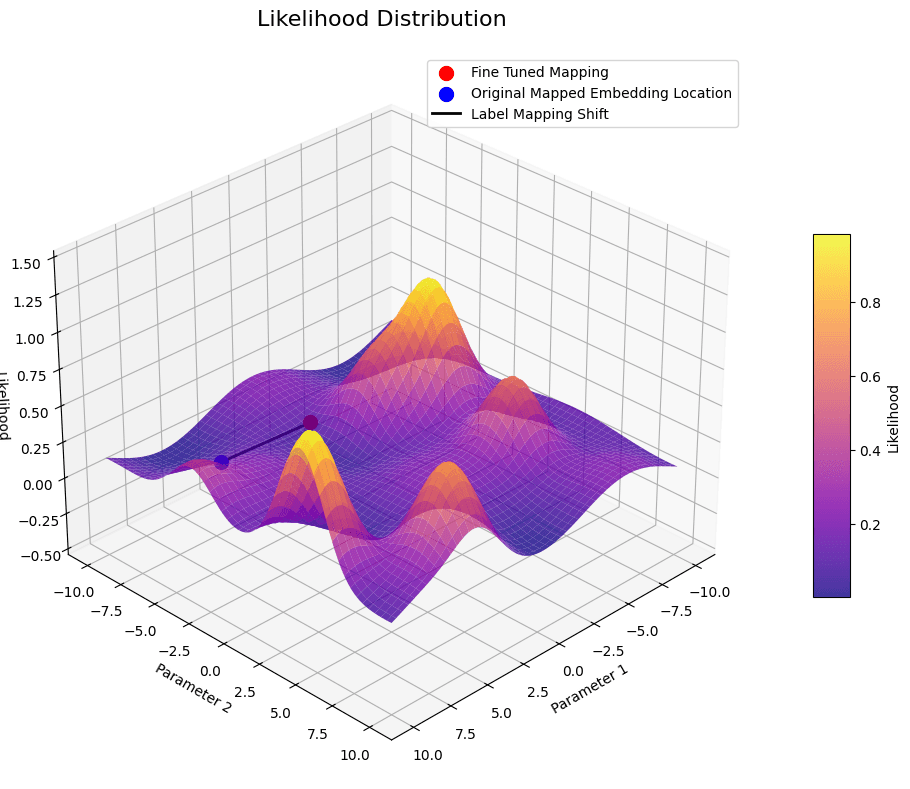
Dreambooth circumvents this problem entirely by not modifying the generative model's weights. Dreambooth instead performs fine tuning on the CLIP encoders by associating an unused token combination with the target image. This has the effect of moving a text-to-image mapping along a target image distribution instead of perturbing the distribution itself. This moves the mapped location to a higher probability region, meaning the generated image is more related to the prompt yet it still leverages the accuracy of the original model's distribution.
Unfortunately, this approach comes with a key drawback. If the target being fine tuned for does not exist in the original distribution the model is trained to cover, the fine tuning will be unable to find an appropriate text to image mapping and generate garbage. For example, if you try to add an anime character to a photorealism model, it fails because there is no concept of an anime character on the manifold of photorealism subjects.